- Blog
- A Comprehensive Analysis of Device Fingerprint Spoofing Techniques
A Comprehensive Analysis of Device Fingerprint Spoofing Techniques
The Evolution of Device Fingerprinting: From Cookies to Spoofing Techniques
Subscribe to the Incognia Newsletter
Device fingerprinting is the process of collecting unique information from a device to create a fingerprint you can use to uniquely identify that device—just like human fingerprints uniquely identify each of us. This information includes a device’s attributes, hardware, software, location, and behavior.
However, as device fingerprinting has become more prevalent, privacy-oriented users and bad actors have found ways to avoid being tracked. One powerful way to disrupt device fingerprinting is to use fingerprint spoofing techniques—techniques that manipulate the information taken in by device fingerprinting technology in order to create a false image of the device.
If you compare it to a real-world human fingerprint, fingerprint spoofing would be like criminals changing the individual whorls on their fingertips to avoid being linked to a crime scene.
Fingerprint spoofing presents fraud fighters with an interesting dilemma: How can you accurately identify individual users when you know that the device data you collect for this purpose may not always be trustworthy?
To understand how to tackle this problem, we have to first understand what these spoofing methods are and how they work.
Key TakeAways
- Device fingerprinting can be a valuable tool in the fight against fraud, but fraudsters can easily spoof legacy device fingerprinting solutions
- Factory resets, third-party tools, and the manipulation of device attributes are just a few fingerprint spoofing methods
- Combining next-generation device intelligence with additional signals like location makes for a more persistent, tamper-resistant device fingerprint
The history of device fingerprinting: then and now
Device fingerprinting technology has its roots in the early 1990s when the Internet was still in its infancy. Initially, cookies were the primary means of tracking users online. As early as 2005, device fingerprinting began to emerge as a more advanced and reliable method for identifying and tracking users.
The advent of Flash and JavaScript around this time paved the way for the growth of device fingerprinting, as these technologies enabled more detailed collection of information about a user's device.
As more sophisticated browsers and devices entered the market, the depth and uniqueness of the available data increased, enhancing the accuracy of device fingerprinting. In 2010, a seminal study by the Electronic Frontier Foundation titled Panopticlick demonstrated how effective browser fingerprinting could be.
With the proliferation of smartphones and tablets in the 2010s, device fingerprinting expanded beyond browsers to include various device-level data points. Today, with the rise of the Internet of Things (IoT), the technology continues to evolve, incorporating data from a plethora of interconnected devices.
However, as device fingerprinting technology evolves, so do the countermeasures fraudsters use to outfox it. Even despite its power and accuracy, the race to keep up with fraudsters shows us that device fingerprinting isn’t the same solution it used to be—at least not when used in isolation.
What are the different device fingerprint spoofing techniques?
As Incognia's Chief Revenue Officer John Lindner has said, "There's a strong need in the market, based on the conversations we have every day, for stronger device fingerprinting. Fraudsters are defeating these defenses by wiping a device, or even factory resetting a device so they appear as a new device."
1. Factory resets
This is by far the simplest method of evading device fingerprint. Performing a factory reset on a device—that is, removing all of its data and restoring it to its original factory settings—allows fraudsters to continue using that device on a particular platform even after it’s been flagged by that platform as being associated with fraud. Traditional device fingerprinting solutions are unable to re-identify devices that have been factory reset.
On most devices, resets are as simple as navigating to a “factory reset” or “erase all data” button in the settings menu. Resetting a device doesn’t require any special tools, resources, or technical knowledge.
2. Third party apps and plugins
Some third party apps can thwart device fingerprinting, particularly when it’s done on web browsers (otherwise known as browser fingerprinting). This is another simple solution for those without much technical knowledge. These apps allow users to select which device or browser attribute data they’d like to change, including font, timezone, screen resolution, and so on.
Though these tools are officially marketed towards privacy-concerned users looking to avoid tracking by advertisers, they can also be incredibly useful for fraudsters looking to avoid accountability.
3. Changing device attributes like device ID
The same sort of device attribute switch-arounds that third party apps can do are also possible for more technically knowledgeable actors to do manually. Because device fingerprinting uses information like IP address, operating system, software versions, apps, and screen resolution to identify devices, manipulating these attributes periodically can help obfuscate a device’s identity.
4. Presenting a false user agent (UA) string
User agent strings help servers determine what type of device and browser users are accessing their app or website from, allowing them to return content appropriately. For example, it would be inconvenient if, every time you accessed a website on your desktop computer, there was a chance that you would accidentally be given the mobile version of the website instead. User agent strings help prevent this by giving the site or app server some information to go off of.
Because of the data it provides about operating system, device type, and browser, user agent strings are also helpful tools for device fingerprinting. When someone spoofs their user agent string, they can provide false information about these attributes and manipulate their data to make it look like multiple requests from a single device actually come from multiple devices.
How does device fingerprint spoofing detection work?
If device intelligence alone can’t always be trusted because of the spoofing tactics detailed above, the best way to make it reliable again would be to introduce a second, tamper-resistant signal to pair it with. Incognia does this by combining device intelligence with location intelligence, to create what we call a Location Fingerprint.
Consider this example scenario: A fraudster is trying to abuse new user promotional discounts on a food delivery app. She’s repeatedly factory resetting her device to change her device ID so that she’ll look like a novel user each time.
If a traditional device fingerprint (including device ID) was the only signal the app had to detect individual devices, there would be little they could do to stop this promo abuse. In fact, they wouldn’t even be able to identify that it was happening; they’d just see what looked to them like many different devices.
However, with the addition of a signal like location intelligence, fraud prevention stakeholders would be able to see based on geolocation data that all this activity was actually coming from the same person on a single device.
By combining signals like GPS, WiFi, and Bluetooth, it’s possible to create a unique image of a device’s location with a false positive rate of less than 1%. This accuracy makes it incredibly reliable for identifying individuals.
In addition to combining traditional device fingerprinting with other signals, platforms can also fight fingerprint spoofing by performing device integrity checks to search for known spoofing apps and plugins. This can help weed out bad actors further upstream in the user journey.
Device fingerprinting is a powerful technology used by apps, advertisers, governments, and even law enforcement agencies to track and identify individuals. However, as bad actors have become more aware of how device fingerprinting works, they’ve also become more aware of its vulnerabilities and how it can be manipulated and evaded.
When device fingerprinting and location intelligence are used in tandem, both signals become much stronger and more persistent. This combination can be a critical advantage in the fight against bad actors who are spoofing fingerprints.
It’s clear that it’s time for traditional device fingerprinting to advance to the next stage of its life cycle. Using device intelligence in combination with other strong anti-fraud signals allows platforms to hold fraudsters accountable and protect their good users from fraud and abuse.
To learn more about how Incognia is advancing device fingerprinting technology on web and mobile, visit our device fingerprinting page.
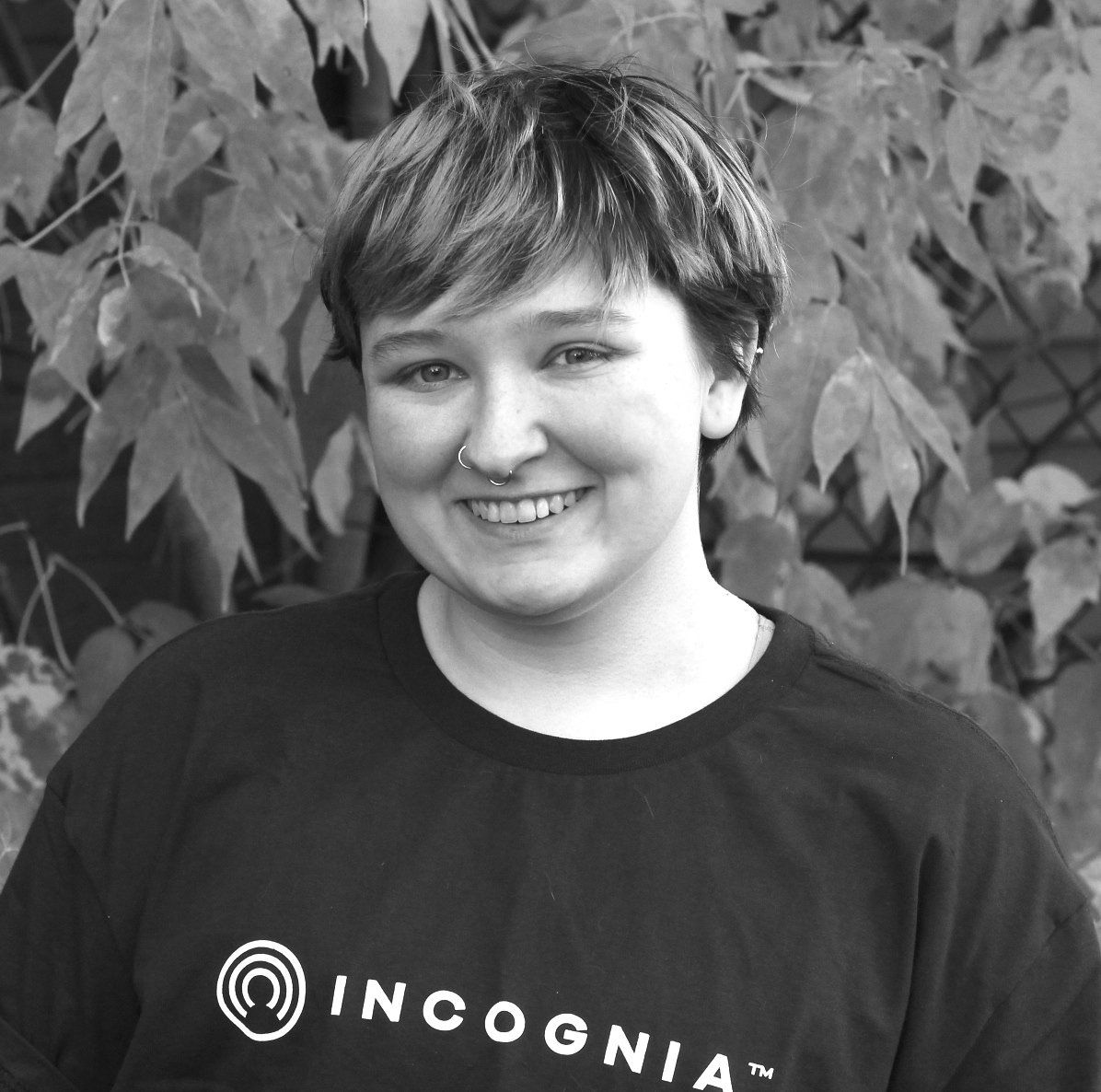