- Blog
- Machine Learning: How Incognia Data Makes the Difference
Machine Learning: How Incognia Data Makes the Difference
In this article, we take a closer look at how Machine Learning drives fraud prevention strategies and explore the benefits it can offer businesses.
Subscribe to the Incognia Newsletter
Machine learning (ML) is a branch of artificial intelligence that’s become more topical in recent years as the technology gains ground in different fields and use cases.
Key TakeAways
- Diverse ML use cases: Machine learning is employed for personalized user recommendations, as well as for detecting and preventing fraud in sectors like insurance, healthcare, and online marketplaces.
- Incognia's approach: Incognia uses tailored models, large first-party data sets, quality fraud feedback, and ongoing model training for improved fraud detection.
- High-quality data significance: Anti-fraud ML models require quality data for supervised learning to stay reactive to evolving attack vectors and maintain accuracy.
The basics of machine learning
ML is a subtype of artificial intelligence. Artificial intelligence (AI) is a broad field that aims to develop intelligent machines capable of performing tasks that typically require human-like intelligence, such as reasoning, problem-solving, perception, and learning. AI involves developing algorithms and models to make decisions and predictions based on input data and feedback; AI systems also adapt and improve their performance over time.
The machine learning subfield of AI focuses on developing algorithms that can automatically learn patterns and relationships in data without being explicitly programmed. In other words, ML algorithms use statistical techniques to find patterns in large datasets and make predictions or decisions based on those patterns.
Machine Learning emerged as the leading AI subfield starting in 2015 with the Imagenet image recognition challenge. In this event, CNN (Convolutional Neural Network) models trained by humans using supervised ML outperformed human capability in recognizing different types of images.
The first prerequisite for meaningful model results is access to large data sets of high-quality data and the ability to leverage a feedback loop wherein humans can help train the models. Said differently, the more data the models have to work with, the better results they can produce. Likewise, the more feedback the models can gain from human interactions, the more they refine their analyses.
Machine learning applications
It’s clear why a machine learning model that creates suggestions based on large pools of data would have various commercial use cases. For example, Netflix uses ML to provide its user suggestions on movies they might like based on their previous watch history and review behavior. Similarly, Amazon uses ML algorithms to analyze buying behavior and suggest different products to each user based on their individual preferences and browsing patterns. Many companies today use machine learning to increase conversions and augment the customer experience.
Machine learning has also been applied to fraud prevention. For instance, one machine learning study in Machine Learning with Applications: Volume 5 found that ML models could be used effectively to predict fraud in property insurance claims. Researchers used real-world data sets from major Brazilian insurance companies in order to determine which machine learning models yielded the best results; deep neural networks and ensemble-based methods outperformed the industry standard method of logistic regression. The study also cited other researchers conducting similar fraud prediction research in the healthcare industry.
These types of prediction models are typically trained with the help of fraud analysts who group the risk assessments made into fraud/non-fraud cases, providing supervised learning for the model. Machine learning algorithms can then help detect fraud by analyzing large datasets in order to identify patterns and anomalies that may indicate increased risk of fraudulent activity.
Incognia is another example of an organization leveraging machine learning as an effective tool for detecting and preventing fraud. However, there are four critical components to Incognia’s machine learning technology that differentiates it:
1. Machine learning models
The CNN (Convolutional Neural Network) and DL (Deep Learning) class of models used in other domains—such as Image Recognition and Natural Language Processing—can also work to predict fraud. However, these are not the best models for fraud prevention because fraud usually represents a very small percentage of the overall transaction volume. Depending on the market vertical, fraud rates vary from a fraction of a percent to a few percentage points. Given this, the data set used to train the ML models is strongly unbalanced in that there are many more good transactions than fraudulent ones.
Incognia was able to achieve better fraud prediction results using algorithms and models tailored to “unbalanced” training sets, such as LightGBM (short for light gradient-boosting machine) and XGBoost (which stands for Extreme Gradient Boosting). These machine learning models have an augmented ability to learn from training sets that have a relatively small percentage of fraud data compared to the total transaction data. Incognia models ”feature maps” containing hundreds of parameters, including device data and location data. This improves the performance of its machine learning.
2. A vast data set
Incognia has access to first-party location and device data collected directly by its SDK from 200M+ devices in over 25 countries globally. The Incognia SDK triangulates locations using several different signals coming from a mobile device to deliver unprecedented precision . Given this data collection method, Incognia’s location data is extremely high quality. For example, user location is precise up to 3m (10ft) which enables the delivery of an identity signal that is about 17x more unique than Face ID, leading to fewer false positives.
3. High-quality fraud feedback
Incognia uses fraud feedback delivered via API by customers to improve its fraud models by providing supervised machine learning. Chargeback prediction and account takeover prediction are the two fraud scenarios that are most improved by these models. While other fraud solutions might use third-party data to train their models, Incognia only uses high quality customer feedback. Using this approach, Incognia is focusing on building reliable models that deliver low error rates when predicting fraud.
4. Continuous model training
Machine learning models must be continuously tuned as new attack vectors emerge to ensure responsiveness. As fraud techniques adapt, the models must be retrained to understand new scenarios. Reliable customer feedback provides the building blocks required to develop effective machine learning models and to retrain the models so they remain sensitive to new attacks.
Machine learning algorithms can help analysts identify fraud, but the datasets used to train them are crucial to their effectiveness: in other words, training an ML model is a “quality in, quality out,” style endeavor. Using high-quality data like direct customer feedback for supervised ML results in a model that is more reactive to the types of fraud being faced by anti-fraud customers in the wild. As fraudsters learn new ways to take advantage of companies, the models used to predict fraud have to keep learning as well. Ultimately, prioritizing high-quality datasets for supervised learning is the best way to produce an effective anti-fraud ML model that can be updated to stay reactive to ever-innovating attack vectors.
Implementing the machine learning models described here, Incognia has successfully stopped chargeback fraud and account takeover fraud for several customers in the food delivery, marketplace, and gig economy verticals.
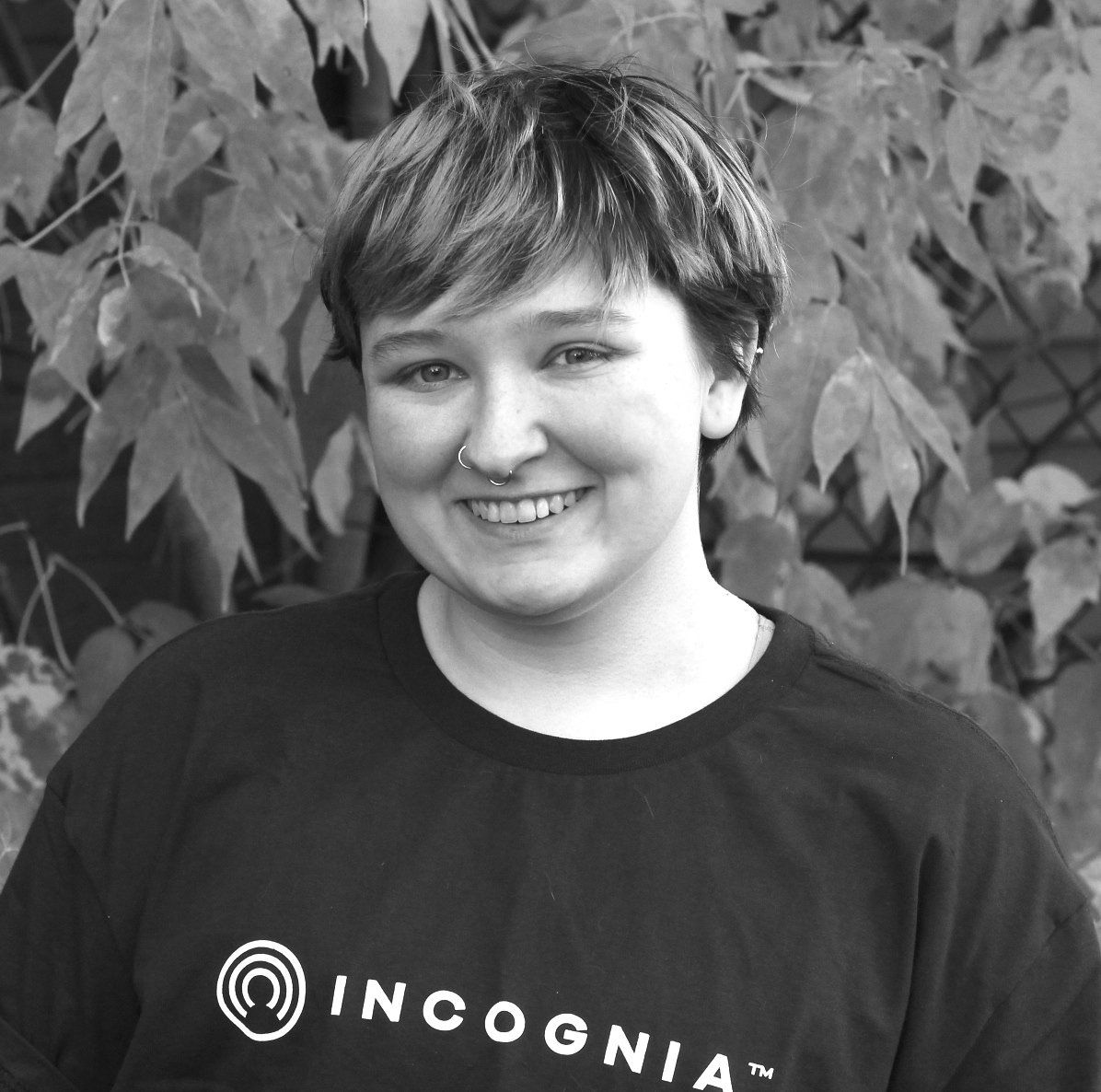